Meet Jane Doe, a loyal customer of XYZ Bank for several years. Recently, Jane has noticed something special happening on her online banking portal. She has been receiving a series of tailored notifications and alerts on specific banking areas such as the following:
- Alerts about the bank services she has not been using, and the benefits of using those services.
- Options to convert some of her large credit card transactions to EMIs.
- Notifications recommending her to use her debit card for transactions to avail cashback.
- An attractive student loan offer based on her daughter’s data who is soon expected to go to college, and so on.
These are all examples of personalized notifications that demonstrate how efficiently this bank has been able to gather customer intelligence around Jane Doe and leverage that intelligence to present her with the products she may need at the time based on her data.
Did you know? The current generation of customers change their banks just after two negative experiences and a whopping 82% of these customers share their experience with others. Data also shows that banks lose 1 out of every 5 customers due to poor experience.
Modern banking and financial services (B&FS) enterprises have realized the pressing need to deliver personalized experiences to customers. For this, the B&FS sector is increasingly adopting advanced personalized recommendation systems. These advanced systems powered by AI technologies are allowing B&FS organizations to efficiently collect, sort, and analyze large volumes of customer data using AI algorithms. This data is becoming instrumental in understanding user preferences, behaviors, and other factors to deliver hyper-personalized customer experiences amidst the backdrop of growing customer expectations and stiff competition from emerging FinTech.
In the first part of our two-series blog post on this topic, we discussed the numerous benefits and best practices of adopting product recommendation systems in banking & financial services. In this article, we take our readers through some interesting use cases of personalized recommendation systems in the B&FS sector.
5 Interesting Use Cases of Recommendation Systems in Banking
There are endless ways in which advanced recommendation engines can be leveraged by B&FS organizations for a competitive advantage. Using these systems, banks can not only offer tailor-made recommendations that cater to the unique needs and preferences of individual customers but also do a lot more to build a strong customer-centered business model. Let’s explore some of the most significant use cases of recommender systems in this context:
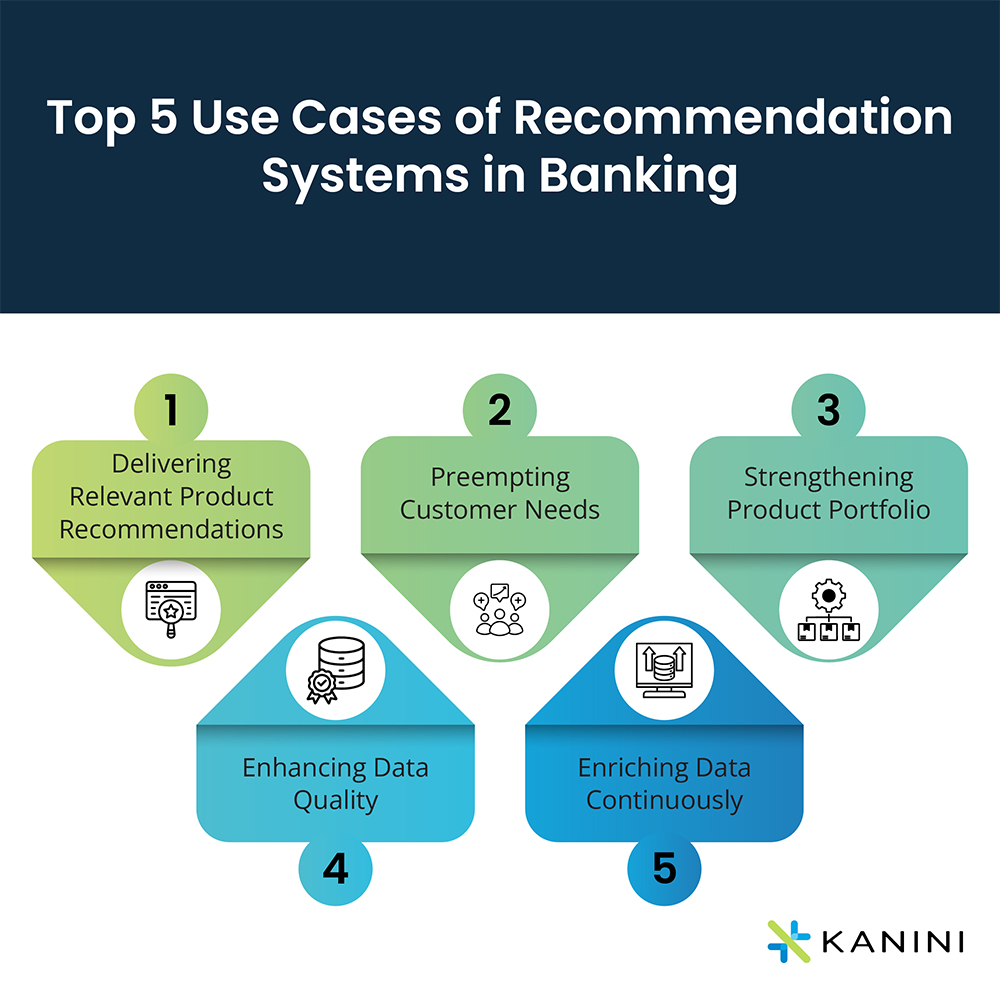
1. Delivering Relevant Product Recommendations
As banking customers, we all receive alerts from our credit card/debit card providers about discount deals on flights, movies, pizzas, salons, and everything that can get us to spend our money. But banks today have come to realize that there is no point in always pushing all products to all customers. Instead, they must evolve as customer-curious enterprises and ensure personalization in their product and service offerings. New-age personalized product recommendation systems are designed to deliver intelligent insights that enable banks to recommend the right products or services to the right customers at the right time.
Using a recommender system, a bank can identify patterns in a customer’s spending habits and customize offers to align them to these transaction patterns. This naturally leads to increased sales and revenue.
Let’s take Jane’s example again. Jane’s spending pattern shows that she often uses her XYZ Bank credit card at a particular taco joint which is immediately followed by another transaction at a nearby ice cream parlor. By analyzing this behavior, XYZ Bank establishes that Jane likes to eat ice cream after her taco meal. The Bank uses this information to locate partnering ice cream parlors, customizes discount deals based on profit margins, and immediately alerts Jane.
2. Preempting Customer Needs
Again, Jane and her daughter Jessica are both frequent users of Bank XYZ’s mobile banking app for their day-to-day transactions. For Jessica, a student with characteristic Gen Z preferences, the app strategically displays her account balance alongside quick-access tools for checking credit scores, budgeting, and splitting bills. This personalized arrangement aligns with Jessica’s likely priorities and preferences.
On the other hand, when Jessica’s mom Jane logs into the same app, she encounters a different configuration. Her initial screen not only shows her account balance but also features her loan balances, investment offers, and insightful stock information.
Both Jessica – representing the Gen Z demographic, and her mom Jane – belonging to a different demographic, witness a tailored interface upon logging in. This is all thanks to the bank’s personalized recommendation system that smartly prioritizes and showcases the banking tools and features that are most relevant and likely to be utilized by each customer. This dynamic adaptation enhances user satisfaction multifold.
3. Strengthening Product Portfolio
Harnessing the power of data science and cognitive automation, a recommendation system enables B&FS organizations to build a robust product portfolio for sustained success. By meticulously analyzing customer behaviors, preferences, and transactional patterns, the bank gains invaluable insights that can be translated into strategic business decisions. The data can be used to refine their existing product portfolio—proactively add or modify offerings to align with customer needs and expectations.
Furthermore, the role of a sophisticated recommendation system goes beyond simple product suggestions, as it can provide a nuanced understanding of customer propensity, allowing the bank to tailor its offerings on an individual level. This degree of personalization enhances customer satisfaction, fostering a deeper connection between the bank and its customers.
4. Enhancing Data Quality
Gartner’s research highlights that poor data quality inflicts an average financial impact of $9.7 million annually on organizations, while IBM reports an annual loss of $3.1 trillion in the US alone due to this challenge. The repercussions of poor data quality, such as slow business growth and loss of potential customers, emphasize the importance of maintaining data quality for effective data commercialization.
Recognizing the critical role of data quality in recommendation systems, companies must ensure that only good-quality data is fed into the recommender system, mitigating the risk of incorrect and incomplete inferences.
Thus, a robust recommendation system not only acts as a catalyst for personalized customer experiences but also safeguards against the substantial financial repercussions associated with poor data quality.
5. Enriching Data Continuously
Financial organizations today recognize the imperative of aggregating data from disparate internal and external source systems. The goal is to construct a comprehensive and valuable data asset, integral in the relentless quest for superior data quality. This data enrichment, when embraced as a continuous process, makes data more current and meaningful, enabling its users to serve customers more efficiently. For this, B&FS organizations must engage in a continuous data discovery exercise. Personalized recommendation systems act as catalysts for data enrichment in banks by leveraging user data, feedback, and behavioral analysis. The continuous learning and adaptation capabilities of recommender systems contribute to the overall enhancement of data and the effectiveness of personalized recommendations in the banking sector.
Adopting Personalization as the Key Strategy
At the heart of every successful banking strategy lies an unwavering commitment to customer satisfaction. The goal is to craft extraordinary customer experiences at every touchpoint, not only to generate revenue but to ensure long-term sustainability. Central to this exceptional customer journey is the principle of personalization, meeting the unique needs of each customer. In this pursuit, recommendation engines emerge as indispensable assets.
For B&FS organizations embarking on this customer-focused expedition, the right guidance and a strategic approach to embracing cutting-edge technologies can help them harness the full potential of modern personalized recommendation systems powered by AI.
Speak with our experts to explore compelling new use cases of personalized recommendation systems and stay at the forefront of industry advancements.
Author
Anand Subramaniam
Anand Subramaniam is the Chief Solutions Officer, leading Data Analytics & AI service line at KANINI. He is passionate about data science and has championed data analytics practice across start-ups to enterprises in various verticals. As a thought leader, start-up mentor, and data architect, Anand brings over two decades of techno-functional leadership in envisaging, planning, and building high-performance, state-of-the-art technology teams.