In today’s competitive business landscape, understanding a customer’s journey has become quintessential for the business growth and success of banks and financial services (BFS) organizations. Thanks to advanced data analytics and artificial intelligence (AI) / Machine Learning (ML) technologies, banking enterprises can unveil and meet the unique needs of their target customers through personalization and skillfully craft their customer success journeys.
Well-built product recommendation platforms, powered by AI and Big Data, successfully fulfill the imperative for personalization in the BFS industry, serving customers according to their preferences while also opening new revenue channels for the organizations.
BFS organizations are increasingly adopting sophisticated product recommendation engines capable of processing large volumes of customer transaction data from various source systems. The fundamental principles of analyzing user behavior, preferences, and historical data to make pertinent suggestions remain constant across different types of recommendation engines. However, product recommendation engines are uniquely designed to propose products that are likely to resonate with potential users, encouraging purchase or engagement. These dynamic systems are built to evolve over time, with user interactions and feedback.
How Do Modern Product Recommendation Engines Match Products and Services to Customer Needs
The strategic adoption of advanced product recommendation engines positions banking and financial services organizations at the forefront of customer-centric innovation, aligning their services to the dynamic shifts in customer expectations and preferences.
Recommendation engines in general are categorized into three main types: a content-based filtering model, a collaborative filtering model, or a hybrid model. Recognizing that each model is built to serve unique business needs, organizations must select the most suitable recommendation engine based on their specific business type and the end objective.
Content-based Filtering Model | Collaborative Filtering Model | Hybrid Model |
---|---|---|
By using the content-based filtering model, banks can leverage the deep insights gained on a particular user’s current preferences to recommend similar products/services in the future. For example, if Customer A has been investing in low-risk, long-term savings bonds, the content-based filtering system recommends similar investment products, such as other bonds or fixed-term deposits with similar low-risk profiles and long-term maturities. | Using a collaborative filtering model, banks can identify patterns in user-item interactions within comparable customer groups to make precise and personalized product recommendations, enhancing the overall effectiveness of targeted offerings. For example, if the credit card transaction data of Customer B and Customer C reveals travel-related expenses and these customers have opted for a travel rewards credit card, then the system automatically offers a travel rewards credit card to Customer D who has a spending pattern that is similar to Customer B and C. | For banking and financial services (BFS) organizations that typically have millions of customers using only a few of their products or services, the hybrid model works quite well. Using the flexible hybrid model which is a combination of content-based and collaborative filtering models, banks can recommend more relevant products and services to their customers. |
What are the Advantages of Using a Product Recommendation Engine in Banking and Financial Services?
A well-built recommendation platform serves as a gold mine of information about a customer’s behavior. This, when done right, can result in making the entire banking enterprise follow a data-driven culture. It is this data-driven culture that helps a bank or any financial services organization in simplifying complex workflows, improving operational efficiency by taking an automation-first approach, and most importantly, empowering its workforce to handle customers with a mindset to improve customer experience.
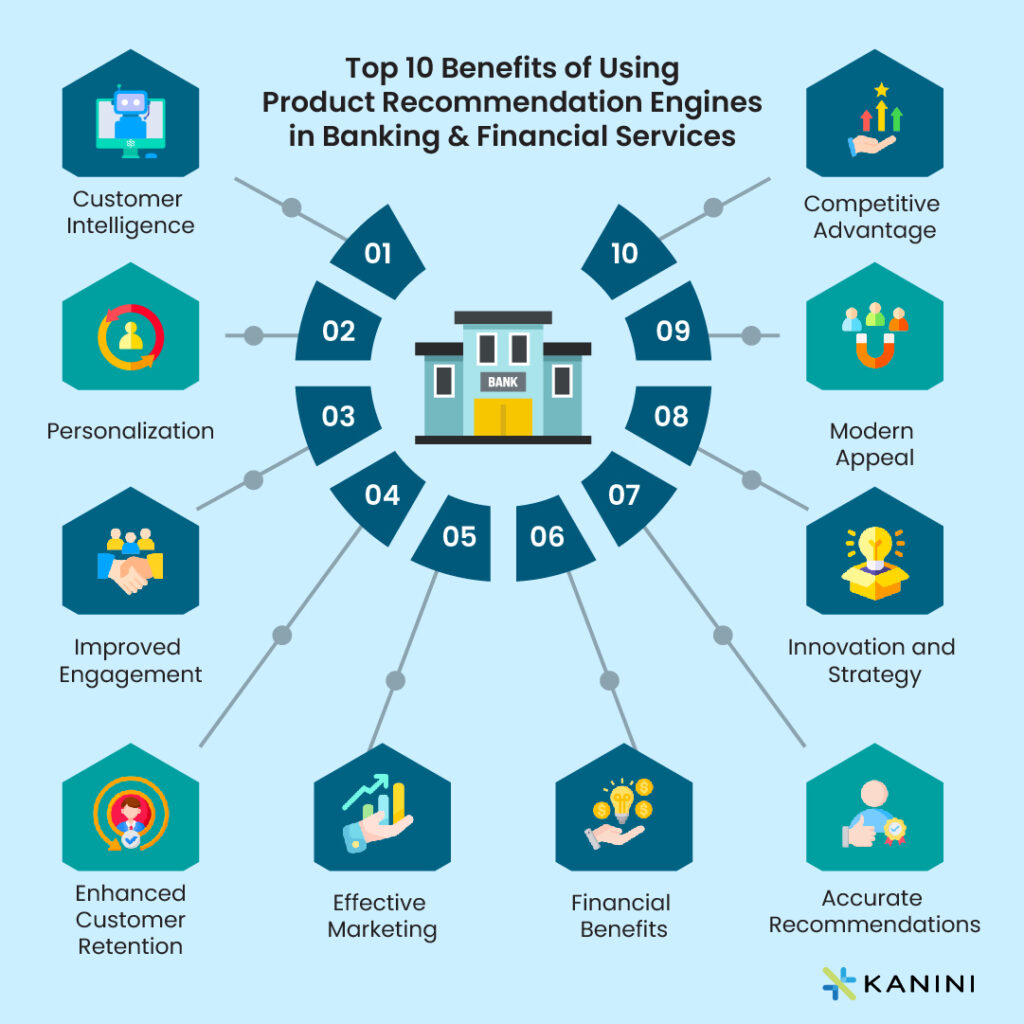
Maximizing the Value of a Product Recommendation Engine in a Banking Enterprise
Building an advanced product recommendation engine efficiently and achieving a faster time to market requires a well-structured approach. Here are some of the best practices to get the maximum benefit from implementing a product recommendation engine in a banking & financial services enterprise:
1. Ensuring Comprehensive Data Collection and Data Integrity
For a product recommender system to work successfully, it needs good-quality data that can be easily accessed. Banks must therefore focus their energies on proactive data collection for deep customer intelligence. From customers’ online/offline banking behavior to their location and even social media activities – every small detail enables the recommender system to understand the customers better and serve them more efficiently.
While comprehensive data collection is critical, data integrity is paramount in building an effective recommendation engine because it relies on accurate and complete data to make informed recommendations. When corrupt and incomplete data is fed into a recommender system, it leads to inaccurate and unreliable insights, causing the bank to make flawed decisions. This can affect customer trust and satisfaction and potentially result in financial and reputational risks for the organization.
2. Upholding Data Privacy, Security, and Governance
The financial data encompassing reference data, transactional data, and social data is inherently sensitive and confidential. While customers appreciate personalized product recommendations, they expect all their data to be handled with care and transparency. The importance of ethical data collection, including obtaining proper consent from customers, especially when it’s personal and sensitive information, therefore cannot be overruled.
Strict adherence to all mandatory compliance and regulations governing data mining and storage is crucial for subsequent analysis and utilization. Safeguarding customer data and complying with privacy regulations becomes easy with robust data governance practices, maintaining data quality, security, and compliance throughout the data lifecycle. These practices enable banks to uphold the accuracy and consistency of data and prevent errors or corruption.
3. Building a Secure and Efficient Data Management Platform
A solid data management foundation is pivotal for efficiently storing and managing the vast volumes of data collected and harnessing the full potential of a recommendation engine. It facilitates seamless data integration from diverse sources and supports data processing pipelines for continuous data cleansing and enrichment.
In this context, cloud-based solutions and data platforms offered by some of the leading solution providers such as Microsoft Azure, Snowflake, and Databricks, offer banking businesses the scalability and flexibility they need for handling big data efficiently along with the added advantage of AI compatibility and real-time data capabilities. Forward-thinking banking enterprises that carefully plan their data modernization roadmap can take advantage of the full potential of advanced product recommendation engines.
4. Adopting a Centralized Dashboard
When enterprises have multiple dashboards for different departments, it creates silos and hinders data flow. Switching to a centralized dashboard that is well-maintained by a technically trained workforce and features a user-friendly UI/UX streamlines data access. Additionally, real-time data visualization and reporting in such dashboards enable quicker responses to changing customer behavior and market trends.
These fundamentals help Banking & Financial Services organizations overcome some of the key challenges they face in adopting recommendation engines such as poor data quality, data sparsity, scalability, cold start problems, and some privacy concerns.
Looking Ahead
With non-banking financial entities and neo-banks breaking traditional banking norms and offering customers a far more flexible service experience, banks today face stiff competition. AI-powered product recommendation engines that empower banking and financial services organizations to deliver a hyper-personalized customer experience, improve their decision-making processes, drive innovation and efficiencies, and build a customer-centric business model, therefore emerging as indispensable assets.
As technology experts, we understand the latest trends and innovations in the rapidly evolving tech landscape. We leverage this knowledge, paired with our extensive experience, to navigate the intricacies of the volatile banking industry.
Speak to us to learn more about how a strategic implementation of a cutting-edge product recommendation engine and data-driven solutions can help you harness customer intelligence and shape the future of your business.
Author
Anand Subramaniam
Anand Subramaniam is the Chief Solutions Officer, leading Data Analytics & AI service line at KANINI. He is passionate about data science and has championed data analytics practice across start-ups to enterprises in various verticals. As a thought leader, start-up mentor, and data architect, Anand brings over two decades of techno-functional leadership in envisaging, planning, and building high-performance, state-of-the-art technology teams.